Rotosolve optimizer¶
Create diagram¶
from lambeq import BobcatParser
parser = BobcatParser()
train_diagram = parser.sentence2diagram('Alice loves Bob')
Create circuits¶
from lambeq import StronglyEntanglingAnsatz, AtomicType
ansatz = StronglyEntanglingAnsatz({AtomicType.NOUN: 1, AtomicType.SENTENCE: 1},
n_layers=2, n_single_qubit_params=3)
train_circuit = ansatz(train_diagram)
train_circuit.draw(figsize=(14, 12))
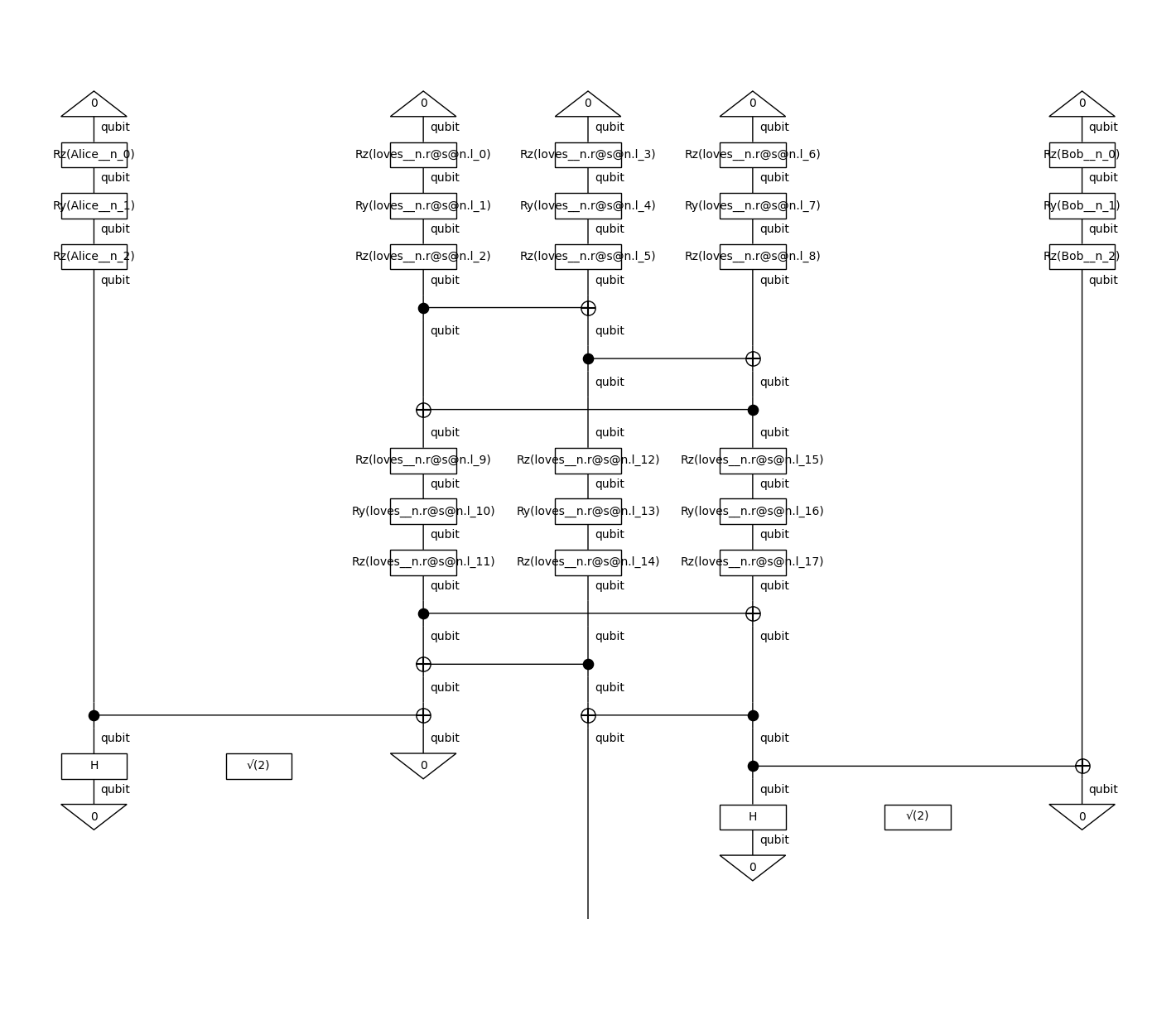
Parameterise¶
from lambeq import NumpyModel
model = NumpyModel.from_diagrams([train_circuit], use_jit=True)
model.initialise_weights()
model([train_circuit]).shape
(1, 2)
Define evaluation metric¶
from jax import jit
@jit
def loss(y_pred, _):
"""The goal is to minimise the expectation value of the Pauli Z operator.
lambeq does not provide functionality to directly calculate expectation
values. Therefore, we need to calculate the expectation value from the
measurement probabilities of the Ket(0) and Ket(1) using their
eigenvalues."""
# 0 state probability
p0 = y_pred[:, 0]
# 1 state probability
p1 = y_pred[:, 1]
# expectation value
exp = p0 - p1 # eigenvalues are 1 and -1
return - exp.mean() # minimise expectation of measuring a 1
loss(model([train_circuit]), None)
Array(-0.21474883, dtype=float32)
from lambeq import Dataset
train_dataset = Dataset(
[train_circuit],
[-1])
Initialize trainer¶
from lambeq import QuantumTrainer
from lambeq import RotosolveOptimizer
from tqdm import trange
EPOCHS = 5
losses = []
for i in trange(20): # calculate results for 100 different seeds
trainer = QuantumTrainer(
model,
loss_function=loss,
epochs=EPOCHS,
optimizer=RotosolveOptimizer,
optim_hyperparams={},
evaluate_on_train=True,
verbose='suppress',
seed=i
)
initial_loss = loss(trainer.model([train_circuit]), None)
trainer.fit(train_dataset, log_interval=12)
losses.append([initial_loss] + trainer.train_epoch_costs)
100%|██████████| 20/20 [00:02<00:00, 8.96it/s]
Show results¶
# get mean and std of the losses
import numpy as np
mean_losses = np.mean(losses, axis=0)
std_losses = np.std(losses, axis=0)
import matplotlib.pyplot as plt
fig, ax = plt.subplots(figsize=(8, 6))
ax.set_title('Training set')
ax.set_xlabel('Epochs')
ax.set_ylabel('Loss')
colours = iter(plt.rcParams['axes.prop_cycle'].by_key()['color'])
ax.plot(mean_losses, color=next(colours));
ax.fill_between(range(len(mean_losses)), mean_losses-std_losses, mean_losses+std_losses, alpha=0.2, color=next(colours));
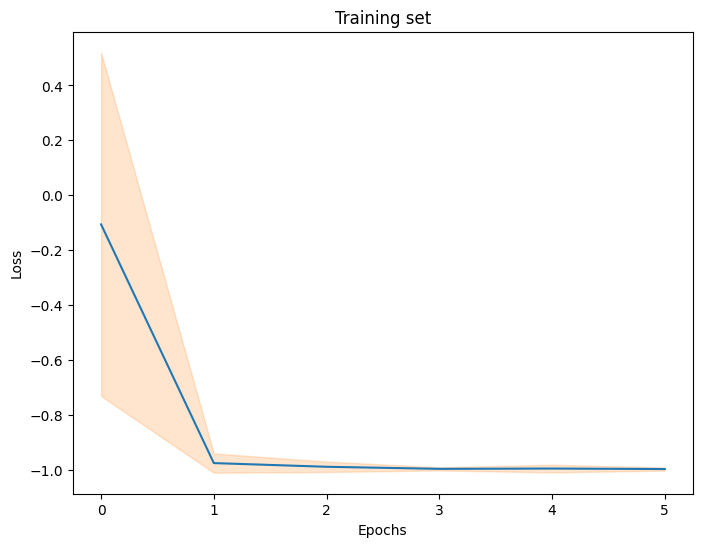
Final output¶
model([train_circuit]).round(3)
Array([[1., 0.]], dtype=float32)